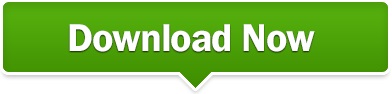
Build predictive models based on structural relationships.Allow domain knowledge to augment model relationship.Use state-of-the-art structure learning methods to understand conditional dependencies between variables.CausalNex aims to simplify this end-to-end process for causality and counterfactual analysis. In our experience, a data scientist generally has to use at least 3-4 different open-source libraries before arriving at the final step of finding the right intervention. counterfactual analysis, and identify the right intervention. We can then use the graph model to assess the impact from changes to underlying features, i.e.Causal relationships are more accurate if we can easily encode or augment domain expertise in the graph model.We believe leveraging Bayesian Networks is more intuitive to describe causality compared to traditional machine learning methodology that are built on pattern recognition and correlation analysis.To estimate the effects of potential interventions using data.ĬausalNex is built on our collective experience to leverage Bayesian Networks to identify causal relationships in data so that we can develop the right interventions from analytics.To allow domain experts to augment the relationships,.
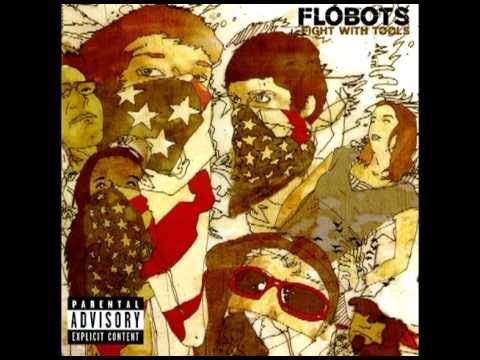
"A toolkit for causal reasoning with Bayesian Networks."ĬausalNex aims to become one of the leading libraries for causal reasoning and "what-if" analysis using Bayesian Networks.
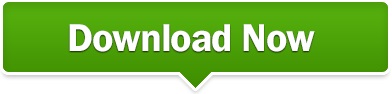